Comparing Chatbots - The importance of training and prompts
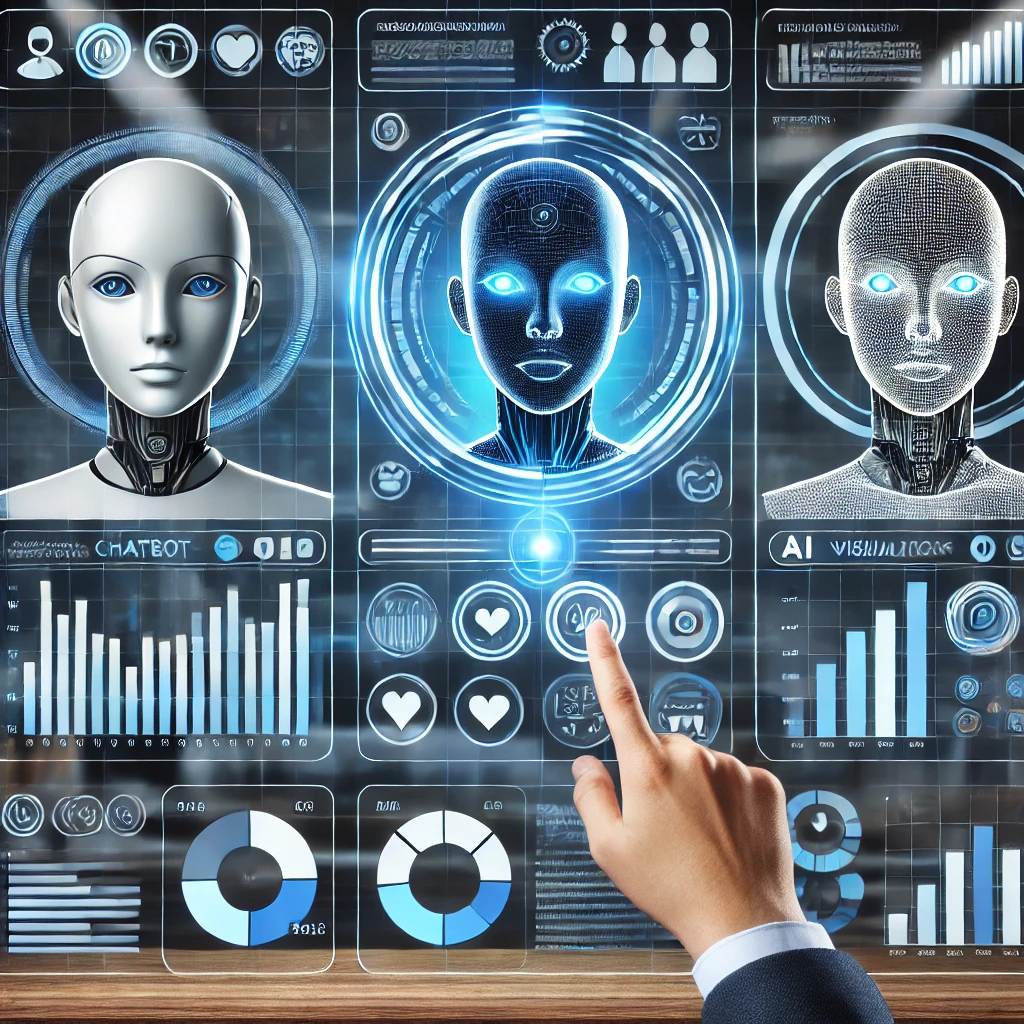
18 November 2024 | Ali Richomme
Comparing Chatbots - The importance of training and prompts
In the last two years, advances in Generative AI (GenAI) have sparked a surge in chatbot applications across various industries, including regulatory compliance. GenAI’s capability to rapidly process information and deliver instant answers has led to the development of regulatory chatbots like AskMax [1] from Cyan Regulatory, Reggie [2] from the Jersey Financial Services Commission (JFSC), and others such as the UK government’s “Gov.UK Chat” [3] and New York City’s “MyCity” [4]. These chatbots use OpenAI’s ChatGPT as their base model, further fine-tuned with custom parameters and data sources to meet specific use cases.
Recently, OpenAI introduced “ChatGPT Search” [5] for its paid users, enabling web searches based on user queries. This new feature highlights the importance of prompt engineering, especially in regulatory contexts where precision is essential. In this article, we explore how the quality of a prompt (being the question or instruction given to the chatbot) affects chatbot responses, using examples from AskMax, Reggie and ChatGPT Search to illustrate how a chatbot’s data sources and the way its parameters are set shape its performance.
What Makes a Good Prompt?
A well-crafted prompt is clear, specific, and contextually aware. When using a chatbot for a regulatory / compliance matter, effective prompts should include details such as regulatory focus (e.g., AML, CDD, data privacy), the relevant jurisdiction, and if possible, a specific scenario. This precision prevents ambiguity and guides the chatbot to provide accurate, relevant responses.
For example, consider a junior employee at a fund administrator asking, “How should I report suspicious activity?” This question is vague, lacking essential context about the regulatory environment, internal policies, and specific procedures. Domain-specific chatbots like AskMax and Reggie handle such questions effectively by providing AML/CFT-specific guidance on SARs, tipping-off rules, and interactions with the FIU. In contrast, a generalist model like ChatGPT might produce a broader, less tailored response, potentially suggesting unrelated actions such as calling the local police or offering generic advice. This highlights the value of well-structured prompts in obtaining accurate and useful information.
In August 2024, a viral incident [6] underscored AI chatbots’ limitations in accurately interpreting prompts. When users asked, “How many 'r's are in the word 'strawberry'?” many chatbots incorrectly answered “two” instead of “three.” This error revealed that chatbots rely on pattern recognition rather than true comprehension, often missing subtle details. The incident emphasizes the importance of precise and prompt crafting and reminds us of the interpretative constraints within AI systems, which can affect their reliability in delivering accurate responses.
Chatbot Parameter Tuning and Document Sources
One of the primary considerations in building effective regulatory chatbots is the selection of document sources and parameter tuning. For instance, when training AskMax, deciding which sources to include was crucial. Should the chatbot recognise “registered person” as synonymous with “supervised person” when cross-referencing the AML/CFT/CPF Handbook and the Codes of Practice?
AskMax and Reggie are both trained on the AML/CFT/CPF Handbook, but they diverge in their supporting documents. AskMax includes legislation such as the Proceeds of Crime Law and the Money Laundering Order, while Reggie focuses on the Codes of Practice. This distinction influences their responses; for example, AskMax can provide legal requirements, while Reggie is better suited to explaining specific terminology within the Codes. Additionally, AskMax has a “human-refined” Q&A feature where Cyan’s experts have written additional information that it can rely on for complex queries or areas where the chatbot would not otherwise able to provide the necessary interpretation.
As an example, when asked “What activities are considered in the sound business practice policy?”:
AskMax lists specific activities, drawing from the Policy as well as the Proceeds of Crime Law and its Refined by Humans page;
Reggie provides a general definition of the Sound Business Practice Policy but omits specific activities.
Whatever sources are used to train a chatbot, anticipating every possible question is challenging, and chatbots sometimes “hallucinate”, generating plausible sounding but inaccurate information. In one notable case in 2023 [7], two U.S. lawyers unknowingly submitted a legal brief containing fictitious cases fabricated by a chatbot. This is one reason why a user should never rely solely on a chatbot’s responses but should double-check the references provided.
It’s also important to understand whether the chatbot’s sources are current. For instance, when asked, “What countries are currently on the D1 list?” both AskMax and Reggie admitted this information was outside their knowledge base. In contrast, ChatGPT Search, with its internet access, could potentially pull in real-time information—an advantage in certain dynamic contexts.
These comparisons illustrate how different data sources and training can impact a chatbot’s ability to provide precise, actionable responses.
Real-World Applications of Chatbots in Compliance
Our compliance software, Beacon [8], integrates customisable chatbot features, using ChatGPT while maintaining strict data security. Users can interact with data directly in fields, documents or forms through natural language prompts such as:
“What controls does this policy stipulate?”
“Create a stacked bar chart of incidents by type and week reported, using brand colors.”
“Draft a root cause analysis document from the information on this form.”
“Recommend action points following this control test.”
This is one way chatbots can enhance compliance processes within software. Other tools, like CaseText CoCounsel [9], assist lawyers with legal research, while LegalZoom [10] helps users draft legal documents. These applications showcase the growing role of GenAI in software.
Conclusion
As regulatory chatbots become integral to the way we work, the quality of prompts and thoughtful selection of data sources will be crucial for delivering reliable, useful responses. While general-purpose models like ChatGPT Search offer a breadth of knowledge, domain-specific chatbots like AskMax and Reggie are essential for compliance professionals navigating complex regulatory environments.
When constructing a prompt, users should consider including details such as the jurisdiction, the industry sector, the subject area (e.g., AML, CDD, data privacy) and perhaps even an outline of the scenario (whilst making sure not to include any names or sensitive data) in order to help guide the chatbot so it can provide a relevant and useful response.
Through constructing effective prompts and being aware of the parameters of different chatbots, users can get the most from these tools to support accurate, nuanced decision-making in an increasingly regulated world.
Citations